Convolutional neural network advances in demosaicing for fluorescent cancer imaging with color–near-infrared sensors
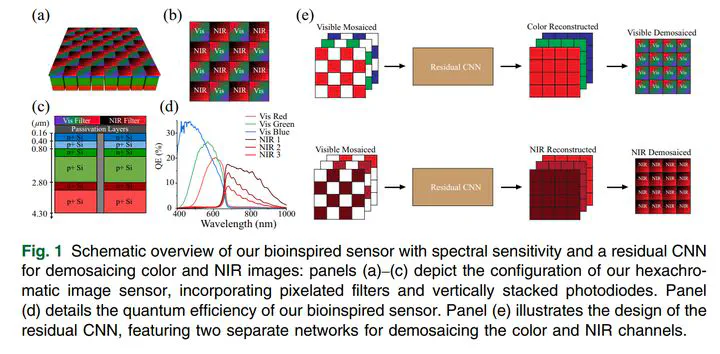
Single-chip imaging devices featuring vertically stacked photodiodes and pixelated spectral filters are advancing multi-dye imaging methods for cancer surgeries, though this innovation comes with a compromise in spatial resolution. To mitigate this drawback, we developed a deep convolutional neural network (CNN) aimed at demosaicing the color and near-infrared (NIR) channels, with its performance validated on both pre-clinical and clinical datasets. We introduce an optimized deep CNN designed for demosaicing both color and NIR images obtained using a hexachromatic imaging sensor. A residual CNN was fine-tuned and trained on a dataset of color images and subsequently assessed on a series of dual-channel, color, and NIR images to demonstrate its enhanced performance compared with traditional bilinear interpolation. Our optimized CNN for demosaicing color and NIR images achieves a reduction in the mean square error by 37% for color and 40% for NIR, respectively, and enhances the structural dissimilarity index by 37% across both imaging modalities in pre-clinical data. In clinical datasets, the network improves the mean square error by 35% in color images and 42% in NIR images while enhancing the structural dissimilarity index by 39% in both imaging modalities.